- Home
- News
- Articles+
- Aerospace
- Agriculture
- Alternate Dispute Resolution
- Banking and Finance
- Bankruptcy
- Book Review
- Bribery & Corruption
- Commercial Litigation
- Competition Law
- Conference Reports
- Consumer Products
- Contract
- Corporate Governance
- Corporate Law
- Covid-19
- Cryptocurrency
- Cybersecurity
- Data Protection
- Defence
- Digital Economy
- E-commerce
- Employment Law
- Energy and Natural Resources
- Entertainment and Sports Law
- Environmental Law
- FDI
- Food and Beverage
- Health Care
- IBC Diaries
- Insurance Law
- Intellectual Property
- International Law
- Know the Law
- Labour Laws
- Litigation
- Litigation Funding
- Manufacturing
- Mergers & Acquisitions
- NFTs
- Privacy
- Private Equity
- Project Finance
- Real Estate
- Risk and Compliance
- Technology Media and Telecom
- Tributes
- Zoom In
- Take On Board
- In Focus
- Law & Policy and Regulation
- IP & Tech Era
- Viewpoint
- Arbitration & Mediation
- Tax
- Student Corner
- AI
- ESG
- Gaming
- Inclusion & Diversity
- Law Firms
- In-House
- Rankings
- E-Magazine
- Legal Era TV
- Events
- News
- Articles
- Aerospace
- Agriculture
- Alternate Dispute Resolution
- Banking and Finance
- Bankruptcy
- Book Review
- Bribery & Corruption
- Commercial Litigation
- Competition Law
- Conference Reports
- Consumer Products
- Contract
- Corporate Governance
- Corporate Law
- Covid-19
- Cryptocurrency
- Cybersecurity
- Data Protection
- Defence
- Digital Economy
- E-commerce
- Employment Law
- Energy and Natural Resources
- Entertainment and Sports Law
- Environmental Law
- FDI
- Food and Beverage
- Health Care
- IBC Diaries
- Insurance Law
- Intellectual Property
- International Law
- Know the Law
- Labour Laws
- Litigation
- Litigation Funding
- Manufacturing
- Mergers & Acquisitions
- NFTs
- Privacy
- Private Equity
- Project Finance
- Real Estate
- Risk and Compliance
- Technology Media and Telecom
- Tributes
- Zoom In
- Take On Board
- In Focus
- Law & Policy and Regulation
- IP & Tech Era
- Viewpoint
- Arbitration & Mediation
- Tax
- Student Corner
- AI
- ESG
- Gaming
- Inclusion & Diversity
- Law Firms
- In-House
- Rankings
- E-Magazine
- Legal Era TV
- Events

Digital Dawn: Data, AI & Future Of Industry
Digital Dawn: Data, AI & Future Of Industry

Digital Dawn: Data, AI & Future Of Industry The Digital Dawn, marked by the convergence of data and AI, is ushering in a new era of industrial transformation. AI, as a new element in production, is revolutionizing the way industries operate Introduction Artificial Intelligence (AI) and Data are at the heart of the technological transformations reshaping industries today. They are...
ToRead the Full Story, Subscribe to 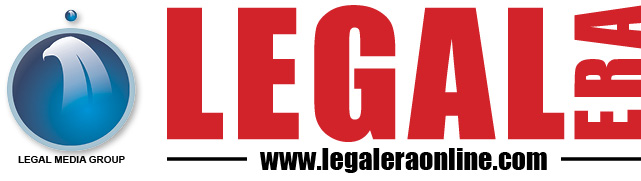
Access the exclusive LEGAL ERAStories,Editorial and Expert Opinion
Digital Dawn: Data, AI & Future Of Industry
The Digital Dawn, marked by the convergence of data and AI, is ushering in a new era of industrial transformation. AI, as a new element in production, is revolutionizing the way industries operate
Introduction
Artificial Intelligence (AI) and Data are at the heart of the technological transformations reshaping industries today. They are the driving forces behind manufacturing industries. These two elements, when combined, have the potential to drive innovation, efficiency, and growth across various sectors. This article explores the symbiotic relationship between AI and data, their practical applications—particularly in manufacturing industry and the future direction of these technologies, including considerations around Data Privacy, Biasness, Accountability issues as well as the risk of model collapse.
Understanding AI and Data
While AI can excel at tasks like data analysis, pattern recognition, and automation, it cannot fully replicate the human capacity for intuition, empathy, and rapid decision-making in complex, dynamic situations.
Artificial Intelligence (AI) refers to the development of computer systems capable of performing tasks that traditionally require human intelligence. These tasks include recognizing patterns, making decisions, and even learning from experience. AI is powered by algorithms—complex sets of rules and calculations—that process data to produce insights and actions.
Data, in this context, is the raw material that AI systems use to operate. Whether it’s numbers, text, images, or sensor readings, data provides the essential input that allows AI to learn and make decisions. In essence, data is the fuel that powers AI, enabling it to generate accurate predictions, identify trends, and optimize processes.

The AI-Data Symbiosis
The relationship between AI and data is one of mutual dependence. AI needs large volumes of high-quality data to learn effectively, while data, often too vast and complex for humans to analyze manually, requires AI to extract meaningful insights.
1. Data Collection and Curation: In any industry, from manufacturing to retail, data is collected from various sources. For example, in a factory producing electronic goods, sensors might track the performance of machinery, recording data on temperature, pressure, and operational speed. The more comprehensive the data, the better the AI can learn and improve its predictive capabilities.
2. Data Processing: Once collected, data must be cleaned and organized. In an automotive plant, for example, data from production lines must be standardized so that AI algorithms can accurately identify patterns—such as predicting when a piece of machinery might fail or when a product defect is likely to occur.
3. Machine Learning: A key aspect of AI, machine learning involves training algorithms on vast datasets to recognize patterns and make predictions. In manufacturing, AI can analyze historical production data to optimize assembly processes, reducing waste and improving efficiency.
4. Actionable Insights: AI’s ability to process and analyze data far surpasses human capabilities, especially in complex environments. For instance, in the automotive industry, AI can analyze data from connected vehicles to predict maintenance needs, improving vehicle reliability and customer satisfaction.
Practical Applications in Industry
AI and data are revolutionizing industries in countless ways. From optimizing production schedules to enabling global logistics, AI is streamlining operations and reducing costs. For example, AI-powered systems can analyze vast amounts of data to predict equipment failures, preventing costly downtime. In logistics, AI can optimize routes, reducing transportation costs and emissions. Additionally, AI-enabled robotics can automate tasks like picking, packing, and inventory management in warehouses.
Beyond operational efficiency, AI is also driving innovation. By analyzing consumer data, businesses can identify trends and tailor their products to meet customer needs. AI can also assist in product design, helping to create more efficient and sustainable products. Moreover, AI can help businesses comply with complex regulations by automating compliance tasks and identifying potential risks.
AI can also play a crucial role in sustainability. By analyzing data, businesses can identify opportunities to reduce waste, minimize carbon footprints, and optimize resource usage.
These advancements are particularly evident in industries such as electronics and automotive, where AI and data are driving significant transformations.
Electronics Industry: The electronics industry is heavily data-driven, from product design to customer support. AI algorithms analyze data from user interactions, allowing companies to refine product features and enhance user experience.
For example, a smartphone manufacturer might use AI to analyze data on how consumers use different features, guiding the design of future models. AI also plays a critical role in quality control, where it can quickly identify defects during the manufacturing process, ensuring that only the highest-quality products reach the market.
Automotive Industry: The automotive industry is undergoing a profound transformation with AI at its core. AI is integral to the development of autonomous vehicles, where it processes data from cameras, sensors, and radar systems to navigate roads safely. Beyond autonomous driving, AI helps manufacturers optimize production lines, manage supply chains, and even personalize the driving experience for consumers. For example, AI-driven systems in smart cars can learn a driver’s habits and preferences, automatically adjusting seat positions, climate control, and even music playlists.
Challenges and the Risk of Model Collapse
The integration of AI and data into industry, while promising, also presents challenges:
- Data Privacy: The vast amounts of data collected by AI systems raise privacy concerns. The more we use technology, the more susceptible we become to data privacy breaches, cyberattacks & other misuses. Industries must ensure that they protect sensitive information and comply with GDPR, DPDPA, IT Act & other related regulation to maintain consumer trust.
- Bias and Fairness: AI systems can inadvertently perpetuate biases present in the data they are trained on. This is particularly concerning in applications like hiring or customer service, where biased AI could lead to unfair treatment. Addressing these biases is critical for the ethical deployment of AI. Industries should ensure that data collected for the recruitment tool is not biased against any specific gender, religion, native, physical characteristics and other such considerations.
- Job Displacement: The automation of tasks traditionally performed by humans is another concern. As AI Robots become more capable, there is a risk of job displacement, particularly in roles that involve repetitive tasks. However, this also presents an opportunity for workforce reskilling and the creation of new types of jobs.
- IPR Issues: AI’s ability to generate content and analyze data raises important questions about intellectual property. For instance, when AI systems generate content—whether it be text, music, design, process or artwork—who owns the rights? Additionally, AI systems often rely on large datasets, some of which may include copyrighted material. This raises concerns about how that data is used and whether it infringes on existing copyrights. Industries must navigate these issues carefully, ensuring that their use of AI and data complies with copyright laws and respects the intellectual property rights of others.
- Model Collapse: One of the lesser-known but significant risks associated with AI is model collapse. This occurs when an AI model, due to continuous training on its own outputs or on increasingly homogenous data, begins to lose accuracy and generalization ability. In industries like manufacturing or automotive production, where AI models are often used for predictive maintenance or quality control, model collapse could lead to inaccurate predictions and faulty operations. To mitigate this risk, it is crucial to ensure that AI models are trained on diverse, high-quality data and regularly updated with new information.
Conclusion
The Digital Dawn, marked by the convergence of data and AI, is ushering in a new era of industrial transformation. AI, as a new element in production, is revolutionizing the way industries operate. While its capabilities are impressive, its success hinges on its symbiotic relationship with human intelligence.
While AI can excel at tasks like data analysis, pattern recognition, and automation, it cannot fully replicate the human capacity for intuition, empathy, and rapid decision-making in complex, dynamic situations. The human touch remains indispensable, particularly in scenarios requiring immediate response, ethical judgment, and the ability to navigate unforeseen challenges. As industries continue to evolve, the optimal approach will likely involve a harmonious blend of AI-driven efficiency and human-centered ingenuity.
Disclaimer – The views expressed in this article are the personal views of the author and are purely informative in nature.